Distributed Machine Learning in Wireless Networks: Challenges and Opportunities
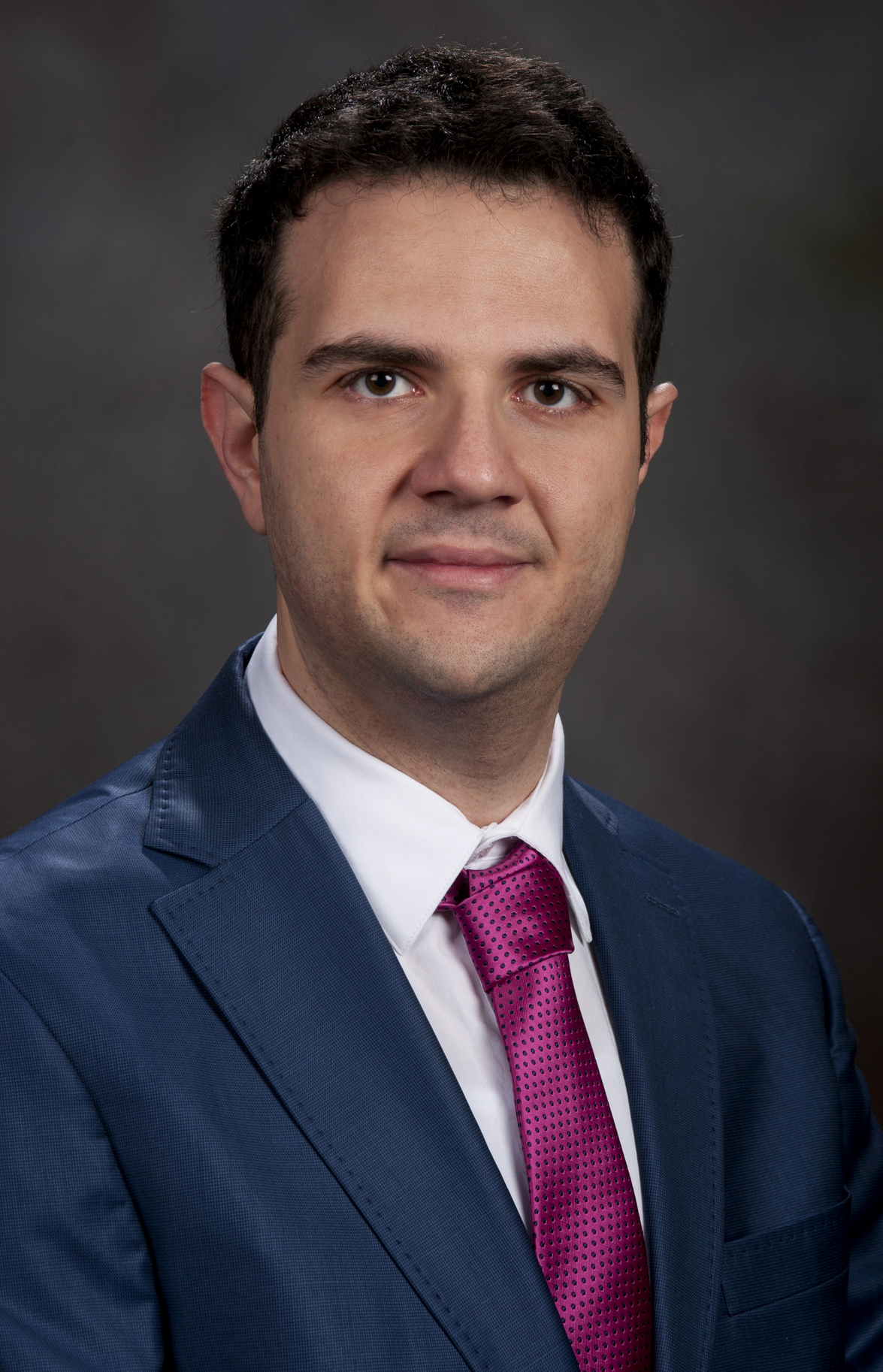
Walid Saad
Virginia Tech, US
Abstract. Due to major communication, privacy, and scalability challenges stemming from the emergence of large-scale Internet of Things services, machine learning is witnessing a major departure from traditional centralized cloud architectures toward a distributed machine learning (ML) paradigm where data is dispersed and processed across multiple edge devices. A prime example of this emerging distributed ML paradigm is Google’s renowned federated learning framework. Despite the tremendous recent interest in distributed ML, remarkably, prior work in the area remains largely focused on the development of distributed ML algorithms for inference and classification tasks. In contrast, in this talk, we focus on two novel distributed ML perspectives. We first investigate how, when deployed over real-world wireless networks, the performance of distributed ML (particularly federated learning) will be affected by inherent network properties such as bandwidth limitations and delay. We then make the case for the necessity of a novel, joint learning and communication design perspective when deploying federated learning over practical wireless networks such as cellular systems. Then, we turn our attention towards the design of new distributed ML algorithms that can be used for generative tasks. In this context, we introduce the novel framework of brainstorming generative adversarial networks (BGANs) that constitutes one of the first implementations of distributed, multi-agent GAN models. We show how BGAN allows multiple agents to gain information from one another without sharing their real datasets but by “brainstorming” their generated data samples. We then demonstrate the higher accuracy and scalability of BGAN compared to the state of the art. We also illustrate how BGAN can be used for analyzing a millimeter wave channel estimation problem in wireless networks that rely on unmanned aerial vehicles (UAVs). We conclude this talk with an overview on the future outlook of the exciting area of distributed ML.
Biography. Walid Saad (S’07, M’10, SM’15, F’19) received his Ph.D degree from the University of Oslo in 2010. He is currently a Professor at the Department of Electrical and Computer Engineering at Virginia Tech, where he leads the Network sciEnce, Wireless, and Security (NEWS) laboratory. His research interests include wireless networks, machine learning, game theory, security, unmanned aerial vehicles, cyber-physical systems, and network science. Dr. Saad is a Fellow of the IEEE. He is also the recipient of the NSF CAREER award in 2013, the AFOSR summer faculty fellowship in 2014, and the Young Investigator Award from the Office of Naval Research (ONR) in 2015. He was the author/co-author of ten conference best paper awards at WiOpt in 2009, ICIMP in 2010, IEEE WCNC in 2012, IEEE PIMRC in 2015, IEEE SmartGridComm in 2015, EuCNC in 2017, IEEE GLOBECOM in 2018, IFIP NTMS in 2019, IEEE ICC in 2020, and IEEE GLOBECOM in 2020. He is the recipient of the 2015 Fred W. Ellersick Prize from the IEEE Communications Society, of the 2017 IEEE ComSoc Best Young Professional in Academia award, of the 2018 IEEE ComSoc Radio Communications Committee Early Achievement Award, and of the 2019 IEEE ComSoc Communication Theory Technical Committee. He was also a co-author of the 2019 IEEE Communications Society Young Author Best Paper and of the 2021 IEEE Communications Society Young Author Best Paper. He is currently an editor for most major IEEE Transactions.
Reliable Federated Learning for Mobile Networks
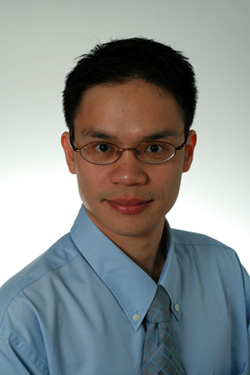
Dusit Niyato
Nanyang Technological University, Singapore.
Abstract.
In this talk, we present an integration of blockchain technology into federated learning for secure and reliable federated learning. The concept of reputation is introduced as a reliable metric for federated learning workers. Based on this metric, a reliable worker selection scheme is proposed for federated learning tasks. Consortium blockchain is leveraged as a decentralized approach for achieving efficient reputation management of the workers without repudiation and tampering.
Biography. Dusit Niyato is currently a professor in the School of Computer Science and Engineering, Nanyang Technological University, Singapore. He received B.E. from King Mongkuk’s Institute of Technology Ladkrabang (KMITL), Thailand in 1999 and Ph.D. in Electrical and Computer Engineering from the University of Manitoba, Canada in 2008. His research interests are in the areas of wireless and mobile networking and distributed computing. He won the Best Young Researcher Award of IEEE Communications Society (ComSoc) Asia Pacific (AP) and The 2011 IEEE Communications Society Fred W. Ellersick Prize Paper Award. Currently, he is serving as editor-in-chief of IEEE Communications Surveys and Tutorials, an area editor of IEEE Transactions on Wireless Communications (Radio Management and Multiple Access), an associate editor of IEEE Transactions on Mobile Computing, IEEE Transactions on Vehicular Technology, IEEE Transactions on Cognitive Communications and Networking, IEEE Wireless Communications, and IEEE Network. He was a guest editor of IEEE Journal on Selected Areas on Communications. He was a Distinguished Lecturer of the IEEE Communications Society for 2016-2017. He was named the 2017-2020 highly cited researcher in computer science. He is a Fellow of IEEE.